We’re kicking off 2023 with a series of blogs about mobility data. This first one takes a step back to remind ourselves what mobility data actually is. Then we’ll reveal what we’ve been working so hard on in 2022!
Sometimes the hype around a source of data can become so incessant and insistent that we don’t even question what’s underlying the enticing outputs.
The dramatic changes in the way we live, work and shop caused by the Covid Pandemic have left the location planning community in desperate need of live or near live information on how places are used. No longer can we rely on gradual changes that we can monitor with a census and some supplementary information from housing building data and monitoring brand openings and closures.
Isn’t there a saying about innovation springing from desperate times? We’ve seen a huge acceleration in the use of mobility data to fill the void, and bring us near real time data on human activity levels. Helping us understand the recovery and ongoing redistribution of visitors to retail places, micro pitch within places, where visitors come from, when they come and who they are. Whilst mobility data is fantastic, as with all data, it’s really important that we understand where it comes from and how best to use it when we make decisions.
Where mobility data comes from
The particular element of mobility data that we are most interested in is the geographic location of a device. This is produced passively, as a by-product if you like, of normal phone usage.
Mobile data can be collected in a number of different ways - varying by collection method, format and context.
The two main sources we use in location planning are app aggregators and network (or telecoms) providers.
Network data comes from the mobile network providers and monitors phone connections to masts and to Wi-Fi, which makes it comprehensive. Historically the downside has been that the reporting cells have been quite large (c.500m) and costs high. However, there is progress on reducing cell sizes and, although costs are likely to remain higher than app data, if the granularity reaches a comparable level, this will be an exciting dataset, especially for venue level insights.
The majority of mobility data used is from app aggregators, but to add to the complexity, all app data is not the same!
The highest quality aggregator data is from first party SDK derived data (Software Development Kit). This is collected directly from a smartphone application without any intermediary parties. The majority of pings are accurate to 20m or less, and it is all real geographical data, with no inferred locations. Volumes can be lower than other sources (described below), but there is greater consistency, accuracy and, for a third party user (like Geolytix), transparency around what the data is.
Other app data mixes in social media and bidstream data to bulk out the data points. Social media and bidstream data don’t collect the device location in the same way as SDK apps (or at all). Bidstream location data for example, is collected when an advertisement is delivered to a mobile device. The location signal can be sourced from the IP address of the device (which has a wide geographical area) or a previously cached location or just a really wrong location when VPNs are used!
Suppliers can be very closed about the types of apps and sources of data they are using. So it’s definitely worthwhile asking a few informed questions about what you’re getting when you buy a product or access to mobility data. And in case you were wondering… GEOLYTIX only use SDK data.
Inherent biases
There are a number of inherent biases in mobile data which vary from provider to provider. Here’s our summary of the aspects that we worry about from a location planning perspective:
- Apps don’t constantly stream data – they will record pings with irregular gaps in time, meaning there can be bias within the sporadic nature of the resulting lat / longs.
- Different apps contribute to the pool of data and this can change over time. We are particularly concerned about how the retail bias of the app mix changes.
- Mobile phone ownership, or not.
- Patterns of mobile phone usage (ie. whether you take your phone out with you or not).
- Using the apps, or not.
- Differentials in all the above biases when it comes to shopping. Do you take your phone to the local shops more or less often than a shopping centre?
BUT it’s absolutely not all doom and gloom!
We can use mobile data ethically, responsibly, and usefully!
Let’s address privacy first of all. Mobility data is not classed as personal data, but the fact that it can be linked to personal data and then provide insights on a person’s behaviour means that we need to treat it with the same level of caution. At GEOLYTIX we only use mobile data from providers that source their data responsibility and are GDPR compliant. We only ever receive and provide anonymised data. For additional protection all our mobility based products and bespoke analysis are provided to our clients at an aggregated level (usually hex grid or Retail Place), with thresholds in place to remove any geographies with small numbers of devices.
Our new UK mobility based products leverage off a flexible mobility data core that has been built internally by our Big Data & AI team. This core allows us to create product and also to do bespoke analysis or cuts of data. We know our customers really value our ability to respond to their specific needs, so we made sure to build in flexibility into our process.
In the mobility data core we compensate for the inherent weakness and biases in the incoming stream of mobile data. There are 6 key processes that are applied within the core:
- Filtering of raw pings to remove unreliable or skewed apps and low productivity devices.
- Dynamic regional population weighting to ensure areas retain consistent relativity, ie. devices and events in Scotland are comparable to devices and events in London.
- As our main use cases are around activity in retail locations, we introduce a retail stabiliser to ensure representation of devices in retail areas is maintained.
- Where pedestrian numbers are required we remove pings occurring in vehicles, at home and at work.
- A modelled interaction surface is used to compensate for the sporadic nature of the event feed.
- Depending on the product or bespoke client requirement we introduce further data sources (such as real footfall surveys) to address any remaining bias in mobile phone ownership or usage.
That’s a whistle stop tour behind the scenes of mobile data. Look out for exciting announcements in coming weeks as we launch our latest mobility based products!
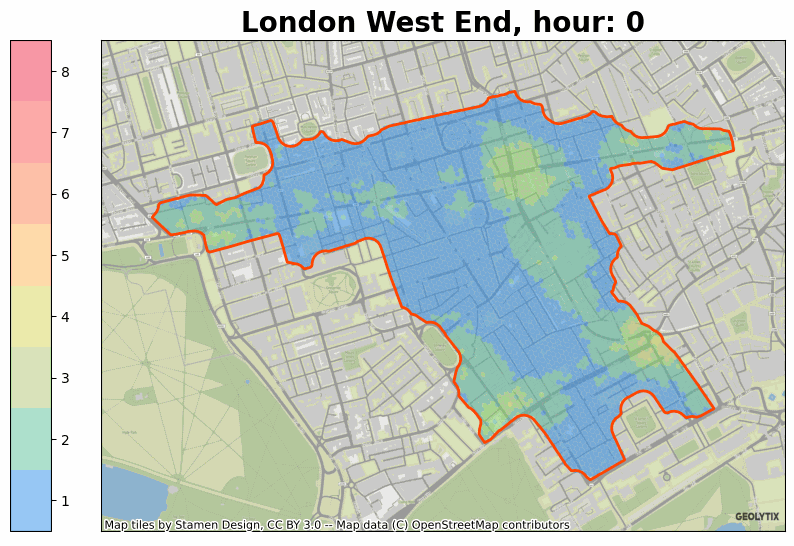
If you’d like to know more about anything raised above then please do get in touch at info@geolytix.com.
Authors:
Samantha Colebatch, Director of New Product Development at GEOLYTIX
Christoph Mulligann, Chief Innovator at GEOLYTIX
Chris Storey, Data Scientist at GEOLYTIX
Alessandro de Martino, Data Engineer at GEOLYTIX
Main Photo by Rodion Kutsaiev on Unsplash